In Recent years dark data has emerged as a critical change component that is shaping the trajectory of artificial intelligence (AI). This hidden diamond mine of untapped value holds immense potential, but its impact on AI is both profound and complex. Harnessing dark data’s potential leaders can significantly enhance the capabilities of AI algorithms. By integrating dark data into machine learning models, organizations can gain deeper insights, uncover patterns, and make more informed decisions.
At times dark data can be a double-edged sword, while the value opportunity within dark data holds promise, its utilization can presents challenges for engineers with limited skillsets. While the potential benefits of dark data are evident, statistics from Forrester Research emphasize the challenges in managing this vast and varied resource. According to Forrester, organizations typically analyze only 12% of their data, indicating a significant gap in effective data management. The complexity of processing and integrating unstructured data poses a considerable hurdle, demanding advanced tools and methodologies.
Dark data is often unstructured, heterogeneous, in some cases unknown and may lack the quality standards required for effective AI integration. The process of cleaning, integrating, and preparing this data for analysis can be complex, requiring advanced tools, an innate sense of perspicacity and expertise. The sheer volume of dark data also poses a logistical challenge, necessitating robust data exploration and data management strategies.
The nature of dark data requires leaders to possess the ingenuity and patience to navigate the shadows. If we were to look at a statistical snapshot of all data collected or all data available for collection we’d find that the majority of it is dark data. According to a report by the International Data Corporation (IDC), dark data represents a staggering 80% of the total data collected by organizations. This includes a multitude of unstructured information, such as emails, documents, and multimedia content, which often remains dormant in archives, unanalyzed and underutilized.
A survey conducted by Deloitte highlights that 94% of respondents are concerned about the potential risks associated with dark data, particularly in terms of privacy and ethical considerations. These concerns are mirrored in the evolving regulatory landscape, with statistics indicating a rising emphasis on data protection laws such as the General Data Protection Regulation (GDPR) and similar frameworks worldwide.
As leaders and their teams delve into the realms of dark data, ethical considerations come to the forefront. Much of this data may contain sensitive information, raising concerns about privacy and compliance with regulations such as personally identifiable information (PII). Striking a balance between extracting valuable insights and respecting privacy is crucial. Responsible AI practices must be implemented to ensure that the use of dark data aligns with ethical standards.
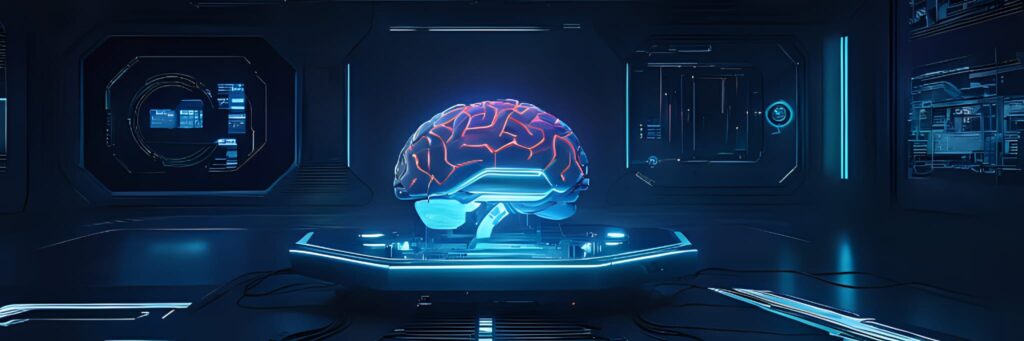
Dark data holds the potential to enhance predictive analytics by providing a more comprehensive view of the data landscape currently available to an organization. The impact of dark data on the overall growth of AI is twofold. First, dark data represents a significant opportunity for AI systems to extract valuable insights and knowledge from unstructured data. By applying machine learning and natural language processing techniques, AI systems can identify patterns and correlations in dark data that would otherwise remain hidden.
Second, the lack of access to this data can hinder the development and training of AI models. AI algorithms rely on large volumes of data to learn and improve over time, and the absence of dark data can limit the accuracy and applicability of AI models.
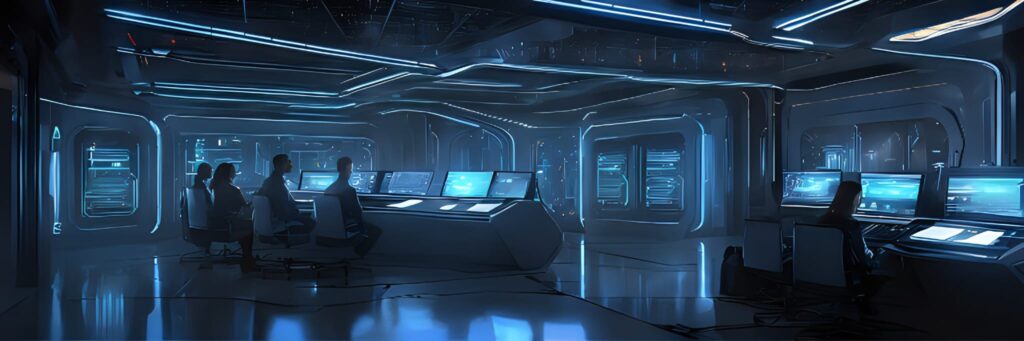
Dark Data can have both positive and negative impacts on the growth of AI. On one hand, dark data can provide a rich source of information for AI algorithms to learn from, which can lead to more accurate predictions and a deeper understanding of customer behavior, market trends, and operational efficiency.
On the other hand, dark data can also present challenges for AI. For example, if the data is unstructured or difficult to access, it may be harder to integrate it into AI models. Additionally, dark data may contain biases or errors that could negatively impact the accuracy of AI models.
Therefore, while dark data has the potential to positively impact the growth of AI, it is important to carefully consider how it is collected, managed, and used to ensure that it does not have unintended negative consequences.
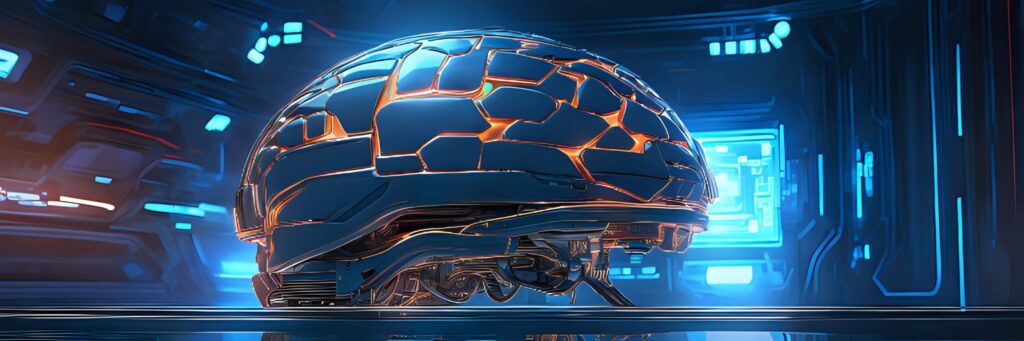
The statistics surrounding dark data and its impact on artificial intelligence has some challenges but are compelling enough for perspicacious leaders to take on the challenge as growth initiatives. As organizations navigate the complex arena of dark data, a focus on addressing data management challenges, data product development, ethical considerations, and cybersecurity imperatives becomes paramount. Statistical evidence, supports the underscoring of the urgent need for leaders and their teams to unlock the full potential of dark data, ushering in a new era of data-driven insights and innovations as AI continues to grow more powerful. The shadows hold not just challenges but a wealth of untapped opportunities for those who poses the pertinacity to illuminate them.
https://t.co/BajEj08dtg#darkdata #technology #AI #machinelearning #blogpost #viralpost #future pic.twitter.com/2csMHA7v5c
— Curtis Thompson (@CurtisLThompson) November 24, 2023