Define and Design
In this module we’ll be starting the process of creating the view of the future deliverable. At this point we have a clear view of the needs for value creation, now we will develop the definition for Dark Data utilization of both internal and external data as well as the exclusion for the Dark Data that is out of scope. This definition and design should be aligned with the overall data strategy, value strategy, and expansion strategy.
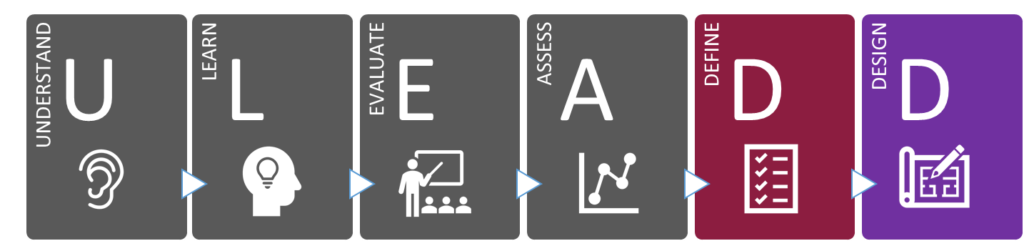
Artifacts that are usually created
- Conceptual Design
- Data Architecture Diagram
- Entity Relationship Diagram (ERD)
- System Architecture
- User interface design
- UX Design
Catalog and Layout a useful Design
Typically, the Captive and Generative Dark Data will deliver a big impact in the MVP and first few release following it. Impact Centered Design (ICD) has a big role in defining and designing a useful end-product.
Step 1
Use the business cases and priorities to identify your plan of attack
Step 2
Catalog all explicit and known data
Step 3
Define the path towards using the captive Dark Data and the Generative Dark Data
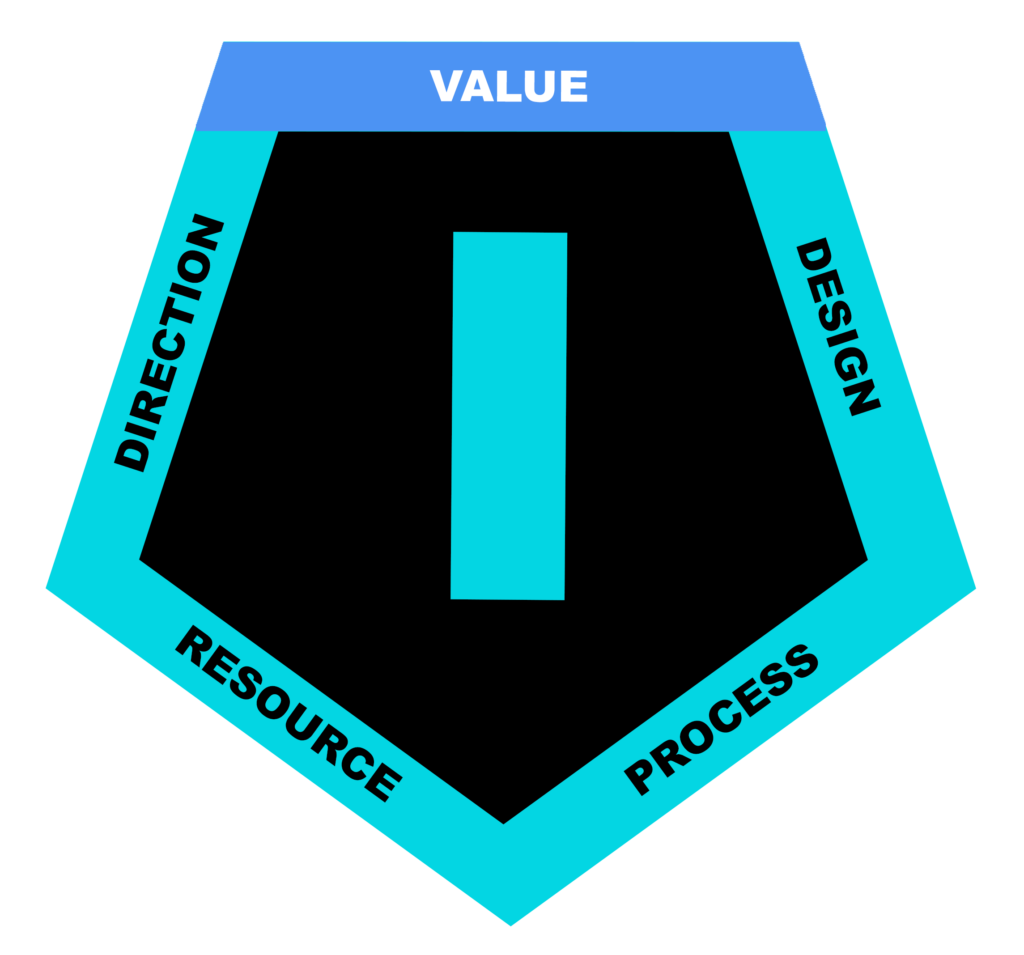
Strategy Defined by Impact-Centered Design
The Overall business strategy should have these three strategic components added to it: data strategy, value strategy, expansion strategy. These three components must be aligned with the overall objectives and direction of the business strategy.
ICD revolves around placing impact at the forefront of project planning. Rather than solely focusing on deliverables, ICD emphasizes understanding the broader consequences of a project on stakeholders, the environment, and society as a whole. By integrating impact assessment into the early stages, ICD provides a comprehensive view that enables decision-makers to navigate uncertainties with clarity. By shifting the focus from outputs to outcomes, ICD aims to create sustainable and meaningful change through a comprehensive understanding of the project’s potential effects.
Traditional management frameworks often focus on tactical attributes of an initiative, thus prioritizing meeting specific objectives, adhering to budgets, and delivering on time. While these aspects are undoubtedly important, they sometimes overshadow the broader context and consequences of the project. This perspective can give way to a general loss of focus on the objectives and outcomes expected from the end deliverable. ICD challenges this tactical-focused perspective by advocating for a more holistic and inclusive approach to planning and execution.
ICD encourages project teams to explore and evaluate the potential impacts that an initiative may have across various dimensions. This involves going beyond the immediate scope of the project and considering the long-term effects on the environment, communities, economies, and other relevant stakeholders. By adopting this broader perspective, teams can proactively uncover hidden risks, opportunities, and unintended consequences that may arise during implementation.
- Data Strategy
- Value Strategy
- Expansion Strategy
The below diagram illustrates how business goes from strategy to delivered value. It depicts the components used to help support the business strategy and the output of the exercised data and analysis. These components are used to help sharpen the strategy and take action. In this process Dark Data has a huge impact along with data quality and the Dark Data hidden within. The ability to take action confidently, efficiently, effectively and in a timely manner is paramount. For a full comprehensive detailing of value strategy development refer to the ReDesign the Box book series.
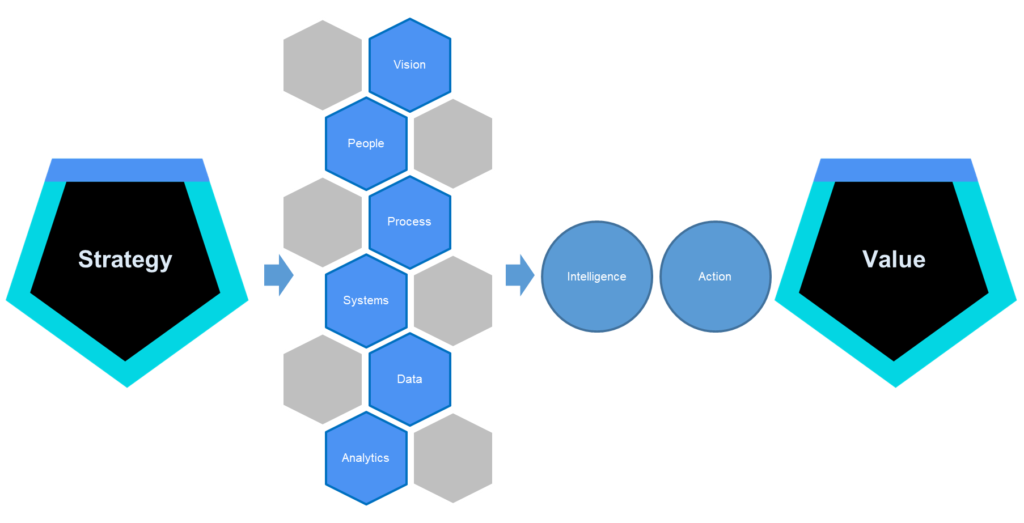
Data Life-Cycle
Given the enormous risk that we discussed in Data Quality issues being a persistent opportunity for improvement in organizations across the world it is important for us to adhere to standard operating procedures that support the prescribed data life-cycle practices. Data lifecycle is a framework that describes the stages that data goes through from its creation to its deletion. It provides a systematic approach to managing data, ensuring that it is accurate, complete, secure, and accessible throughout its lifespan.
- Planning: This stage involves defining the purpose of the data, identifying the data sources, and determining the data requirements.
- Collection: In this stage, data is gathered from various sources and stored in a database or other storage medium.
- Processing: Data is processed to transform it into a usable format, such as cleaning, transforming, and integrating it with other data sources.
- Storage: In this stage, data is stored in a secure and organized manner, ensuring that it is protected from unauthorized access, theft, or loss.
- Analysis: Data is analyzed to gain insights and make informed decisions, such as performing statistical analysis, data mining, or machine learning.
- Presentation/Share: In this stage, the results of the analysis are presented to the relevant stakeholders in a clear and understandable format.
- Archiving: Data that is no longer needed for active use is archived for long-term storage.
- Purge/Deletion: In this stage, data that is no longer needed is securely deleted to ensure that it does not pose a security risk.
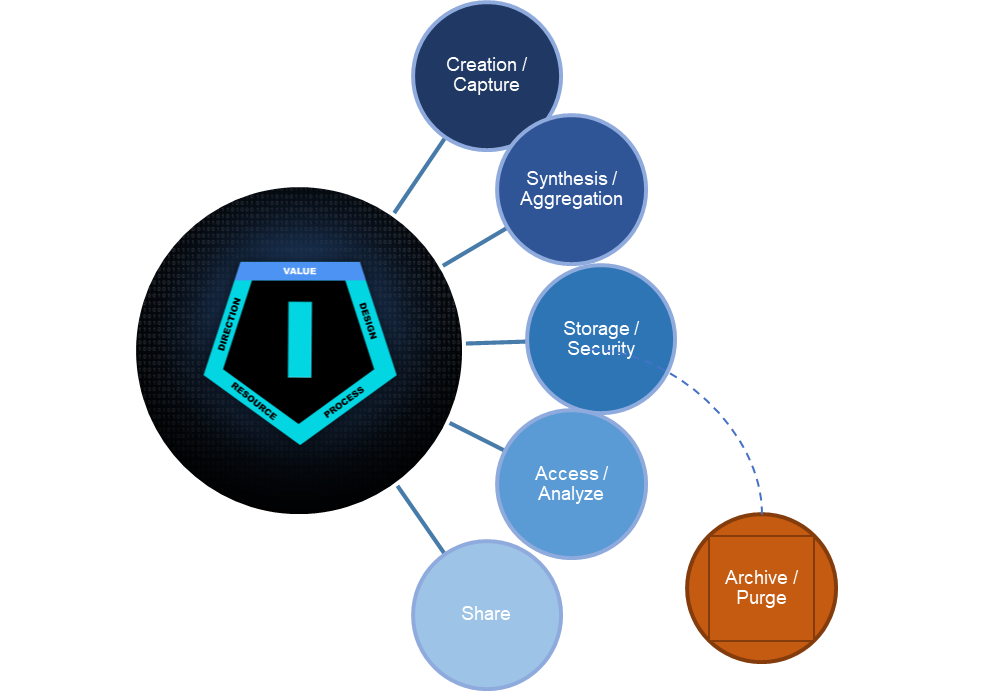