Data granularity is a measure of the level of detail in a data structure. In time-series data
We see the most opportunity for impact from data granularity in the 2nd tier (Generative Data) of Dark Data.
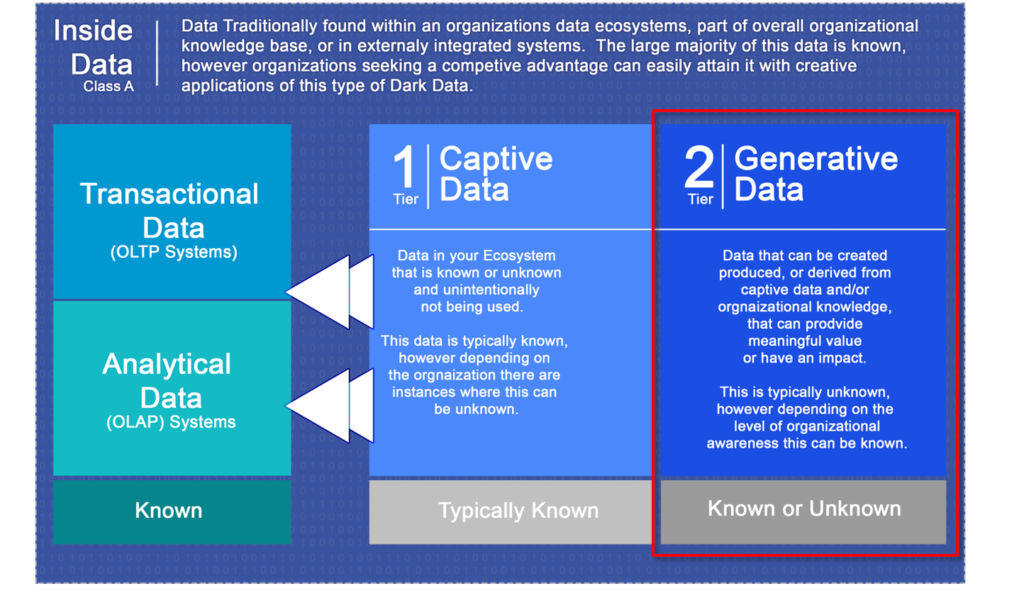
To position yourself for success with Dark Data, you must be both PRAGMATIC and IDEALISTIC.
Benefits of ULEADD
- Clear framework for discovery
- Standardized approach to problem-solving
- Helpful strategies to navigate cultural challenges
- Flexibility for personal touch
It can be difficult to get an organization to see the value in granularity because of the amount detail and work involved in ensuring data is structured in a granular fashion. Typically, organizations want to deliver data solutions in a minimalist manner which causes many organizations to rely on summarized or grouped sets of data that can’t be unwound when deeper insight is needed. This is usually the price organizations pay for simplifying or narrowing focus too soon. Organizations must find the optimal balance between speed and granularity, furthermore they must find the balance between building for scale and speed. Building scale usually requires a commitment to granularity from a data perspective. A universal fact that is persistent in anything that we do especially things in the technical realm is that there are consistently unknowns, even our knowns have unknowns.
This means that we must begin with an understanding of the needs at a granular level then work our way up to the solution. This is more of an art than a science, which is why it is best to use the ULEADD process to work your way into the Define and Design phases of any initiative.
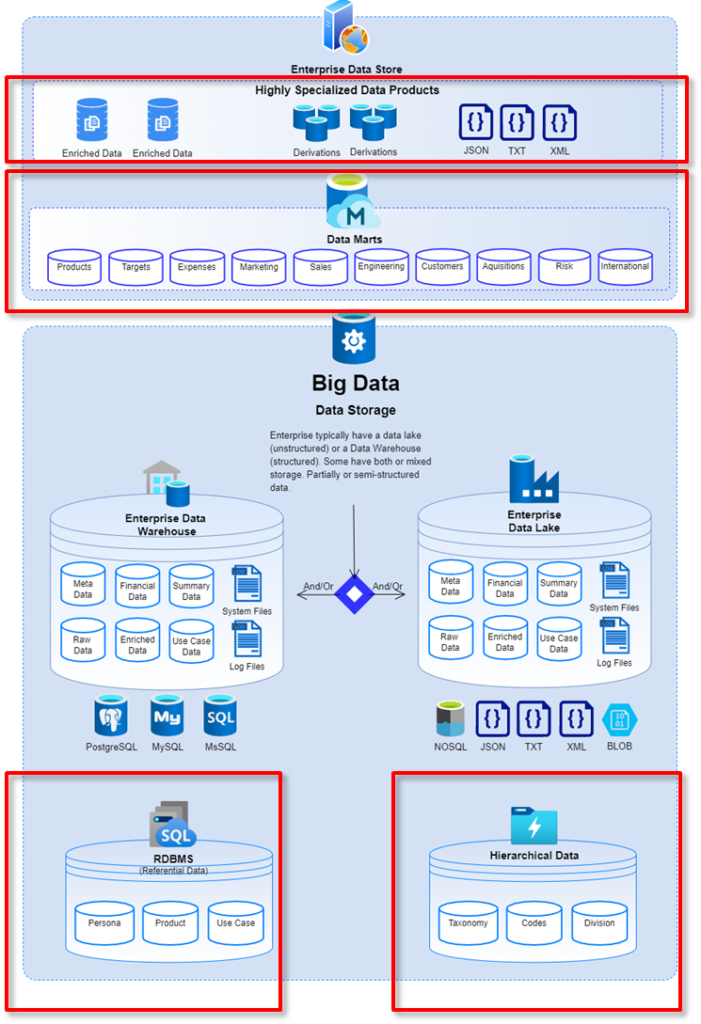
The above diagram is a call out of the generic logical design. the red squares at the bottom demonstrate that the granularity in the supportive historical and referential data has an enormous impact on the overall output of the data as it flows through the warehouse or lake.
Outlined in red at the top is the data in the data marts and in the specialized DILs that store our near end-user data products. This data is dependent on the granularity and structure of the referential data. The foundational data also supports the data marts and DILs through the methods in which the data transformations happen at various stages throughout the data journey. In this scenario the resulting data products can be extremally useful only if the underlying systems have been constructed in such a way where the data scientist & analysts can easily manipulate and scrub through the resulting visuals created by the data products.
Additionally, the decision-makers will obtain greater value dependent upon how much value the data scientists and analyst are able to derive. The synergistic effects of granularity, data products and rapid development of meaningful analysis is fundamental to value creation.