DAMA International
(Data Management Association)
Data Management is the development, execution, and supervision of plans, policies, programs, and practices that deliver, control, protect, and enhance the value of data and information assets throughout their lifecycles.
Definition extracted from the DMBOK (Data Management Body of Knowledge)
The 11 functional areas of Data Management center around Data Governance. For in-depth info on Data Management consult the DMBOK written and published by DAMA international. they are the leading authority on Data Management. the 11 functional areas are illustrated in the diagram below.
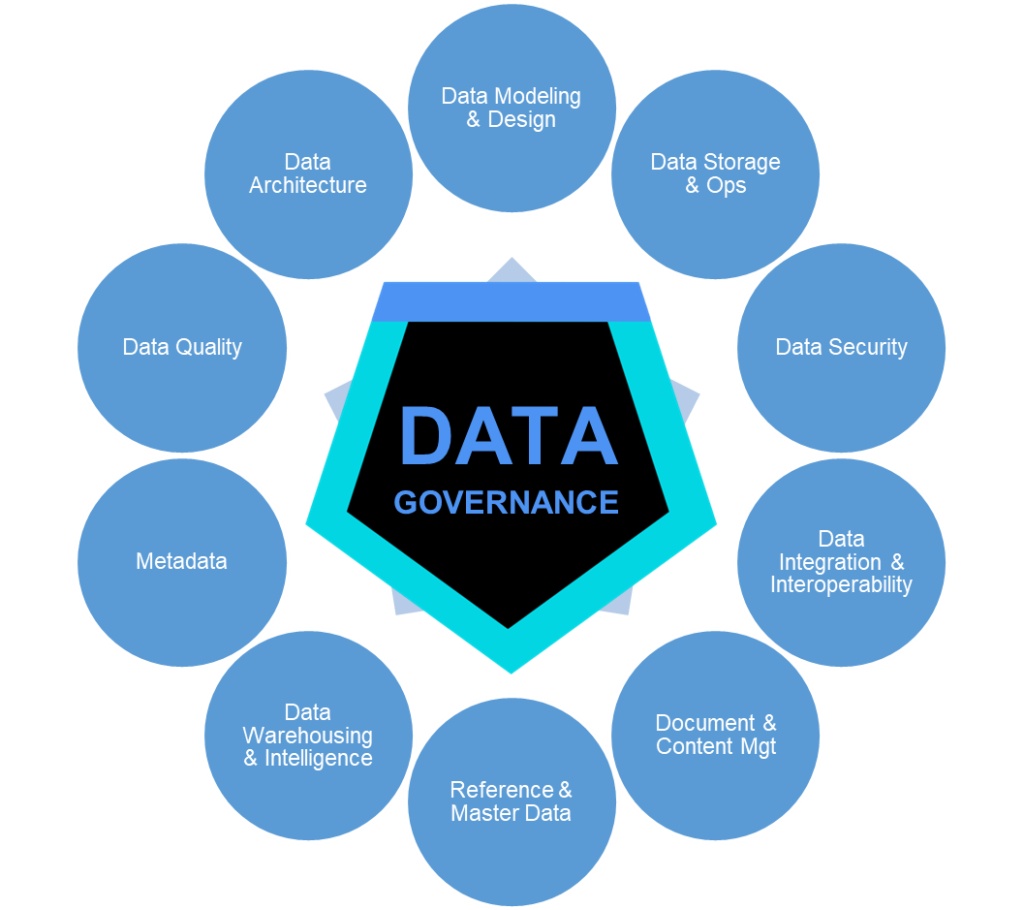
- Data Architecture
- Data Modelling and Design
- Data Storage and Operation
- Date Security
- Data Integration and Interoperability
- Document and Content Management
- Reference and Master Data
- Data Warehousing and Business Intelligence
- Metadata
- Data Quality
- Data Governance
Data Management is critical in facilitating the four V’s of Big Data and the Dark Data that not captured or lost.
Volume | Variety | Voracity | Velocity
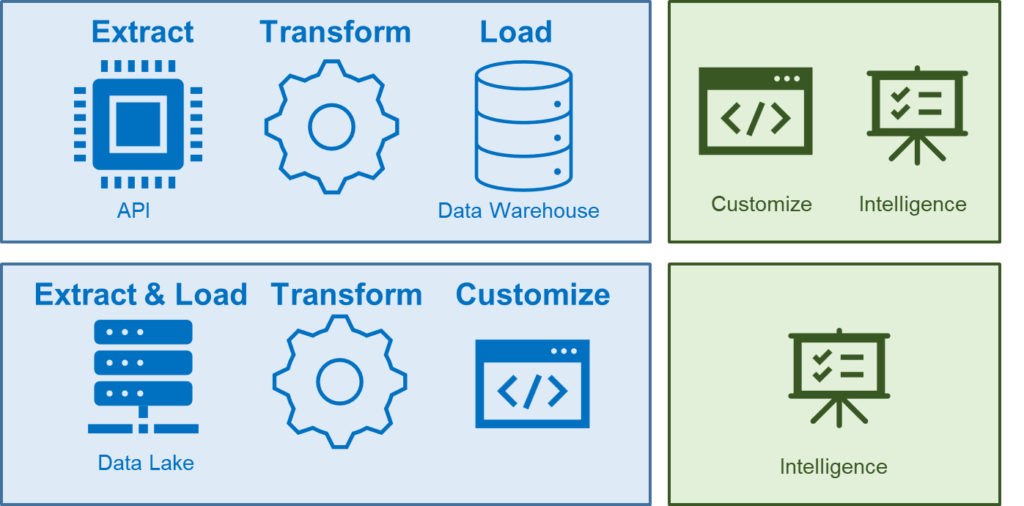
MDM helps to extract value from Dark Data in a few ways.
- Identifying and Cataloging Dark Data: MDM solutions can help organizations identify and catalog dark data, which is data that is unstructured or unmanaged and therefore difficult to use for analytics. By identifying and cataloging this data, MDM solutions can help organizations understand what data they have and where it is located.
- Enabling Data Integration: MDM solutions can enable data integration across multiple sources, including dark data sources. By integrating dark data with other data sources, organizations can gain a more complete view of their data, which can help drive insights and inform decision-making.
- Standardizing Data: MDM solutions can help standardize data across the organization, including dark data. By standardizing data, organizations can ensure that their data is consistent, accurate, and reliable, which can help improve the quality of analytics and decision-making.
- Enabling Data Governance: MDM solutions can also enable data governance, which is the process of managing the availability, usability, integrity, and security of the data used in an organization. By implementing data governance practices, organizations can ensure that their dark data is properly managed and used, which can help reduce the risk of errors and improve the accuracy of analytics.
Master & Reference Data
- Master data is the data shared by multiple systems, applications, and processes; furthermore, it sets a base context for shared attributes across an enterprise.
- Reference data is used to categorize and add context to data, enrich data, and create data in combination with other data fields, attributes or meta data.
Master Data Management (MDM) is highly critical to Fortune 500 companies, as these organizations deal with a massive amount of data and need to ensure that the data is accurate, consistent, and reliable. MDM is a discipline that focuses on creating a single, trusted view of an organization’s critical data, such as customer data, product data, or financial data, by integrating data from multiple sources and maintaining its accuracy and consistency over time.
Example of Master, Reference & Transactional Data
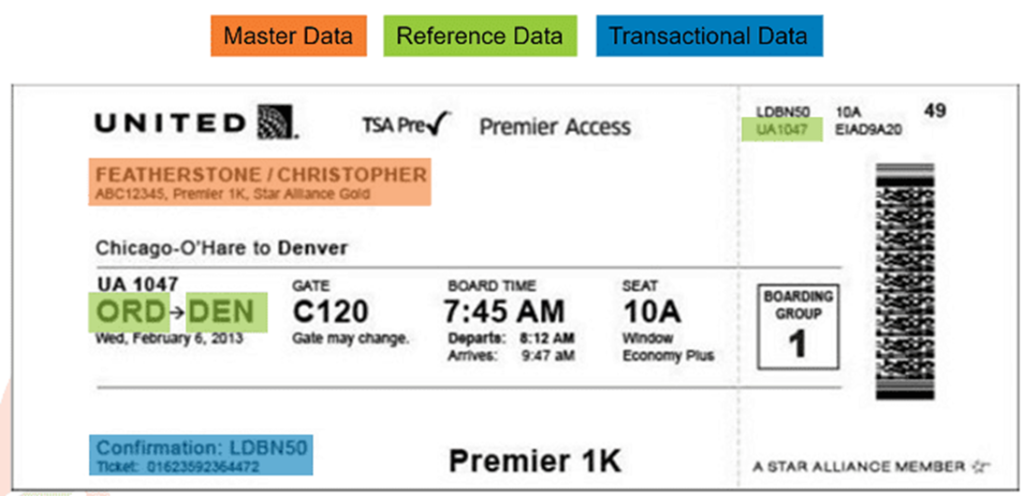
Cost of Poorly Designed Reference Data
If the reference data is poorly designed, inaccurate, or inconsistent, it can lead to a range of problems that can impact the organization’s bottom line. The impacts can be expansive and include things such as: increased operational costs, poor data quality, invalid transactions, data duplicity, synchronization impacts, reduced efficiency, compliance impacts, and missed business opportunities.
In large organizations, data is often stored in multiple systems, applications, and databases, which can result in inconsistencies and errors. Without a solid MDM strategy in place, data may be duplicated, outdated, or inaccurate, leading to poor decision-making and missed opportunities.
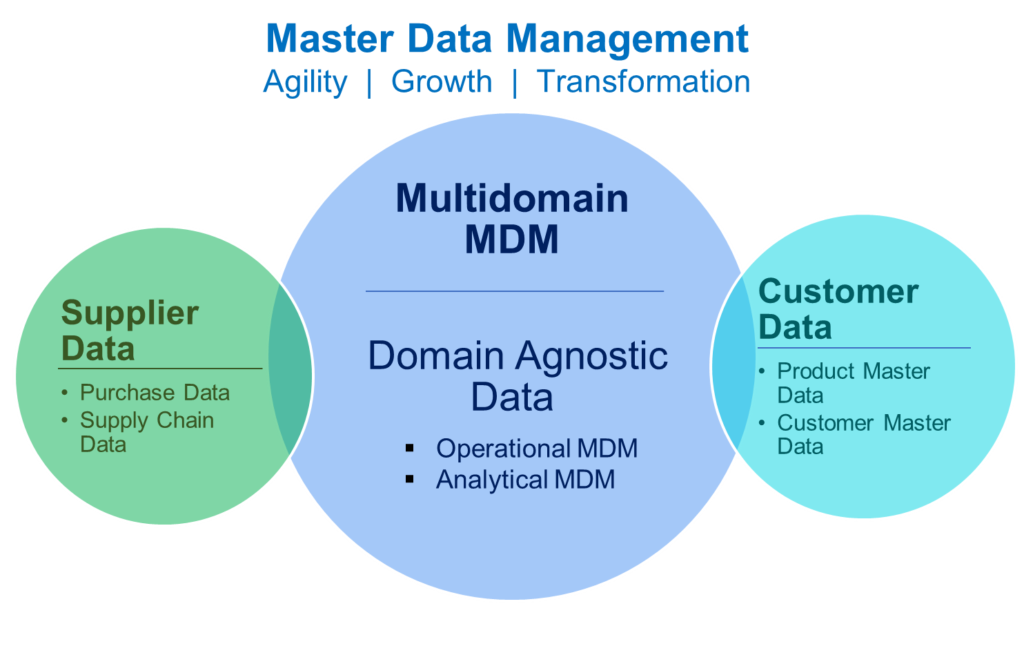
Fortune 500 companies recognize the importance of having accurate and reliable data to drive their operations and remain competitive in their respective industries. MDM enables them to have a single, accurate view of their data, which in turn helps them make better-informed decisions, improve operational efficiency, and enhance customer experience. As a result, Fortune 500 companies are heavily investing in MDM solutions and technologies to achieve their strategic goals and remain ahead of their competition.
Data quality management provides a context-specific process for improving the fitness of data that’s used for analysis and decision making. The goal is to create insights into the health of that data using various processes and technologies on increasingly bigger and more complex data sets.
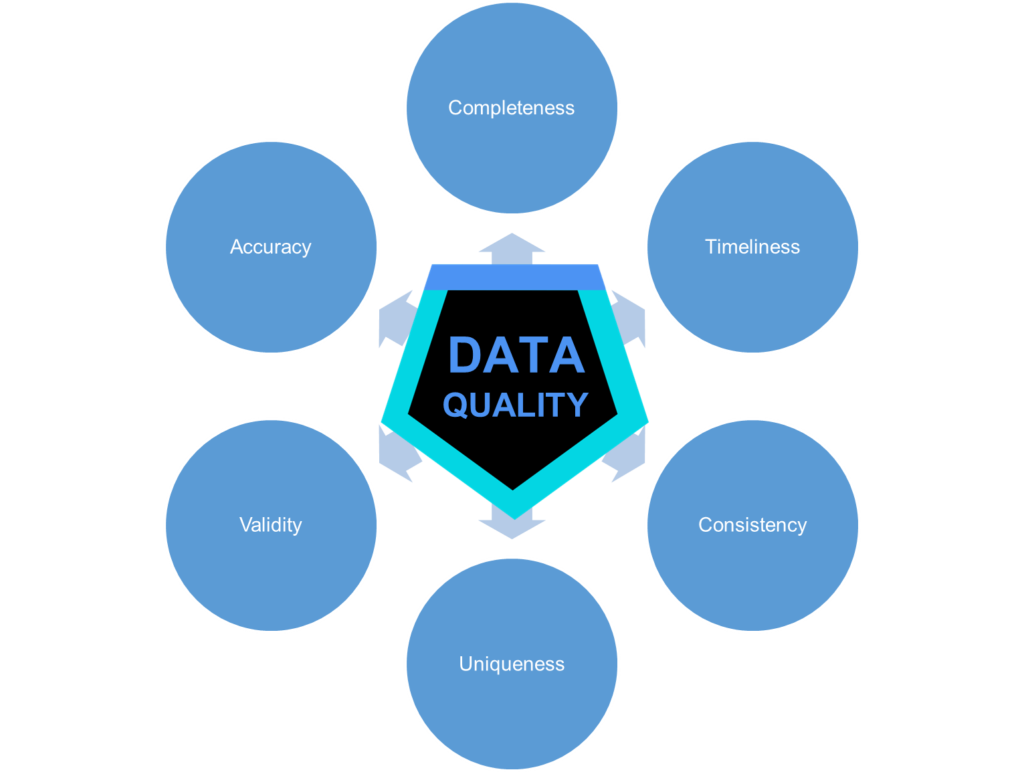
Dark data can have a significant impact on data quality management (DQM) in several ways:
- Incomplete Data Sets: Dark data can often represent a significant portion of an organization’s data, and this data can be unstructured, unmanaged, and difficult to access. This can lead to incomplete data sets, which can impact the accuracy and completeness of DQM processes.
- Data Bias: Dark data can also introduce bias into DQM processes. For example, if an organization only uses data from certain sources or data that is easily accessible, this can create a biased sample that does not accurately represent the entire data set.
- Inconsistent Data: Dark data can also be inconsistent with other data sets, making it difficult to integrate and analyze. This can lead to inconsistencies in DQM processes, which can impact the accuracy and reliability of results.
- Security Risks: Dark data can also pose security risks, as this data is often unstructured and unmanaged. This can make it difficult to protect sensitive information, such as personally identifiable information (PII) or confidential business data.
To mitigate the impact of dark data on DQM, organizations should implement data governance practices to ensure that dark data is properly managed, integrating dark data with other data sources, and using analytics tools to identify and address data bias and inconsistencies. By doing so, organizations can improve the accuracy and reliability of their DQM processes and ensure that they are making informed decisions based on high-quality data
