Standardizing the data enrichment process can help ensure consistency and accuracy in the resulting data.
Some of the steps we can take to standardize the data enrichment process:
- Define clear objectives: Before beginning the data enrichment process, it is important to define clear objectives for what you hope to achieve through the process. This will help guide the selection of data sources, the types of data to collect, and the methods used to enrich the data.
- Establish a clear data enrichment methodology: Once you have defined your objectives, you should establish a clear methodology for enriching the data. This may involve identifying specific data sources to use, establishing criteria for selecting data to enrich, and developing a process for collecting and integrating the enriched data.
- Use consistent data formats and standards: To ensure consistency in the enriched data, it is important to use consistent data formats and standards throughout the enrichment process. This may involve using standard data dictionaries or data schemas, as well as establishing data quality criteria to ensure that the enriched data meets your organization’s standards.
- Document the enrichment process: To ensure consistency and transparency in the data enrichment process, it is important to document the process and any decisions made along the way. This documentation should include details on the data sources used, the methods used to enrich the data, and any data quality issues that were identified and addressed.
- Monitor and evaluate the process: Finally, it is important to monitor and evaluate the data enrichment process over time to ensure that it continues to meet your organization’s needs and standards. This may involve tracking key performance indicators (KPIs) such as data accuracy, completeness, and timeliness, as well as regularly reviewing and updating the enrichment methodology as needed.
Taking these steps can help to reduce failure. Some commonly encounter failures are: incorrect conclusions, misinterpreted data, poor messaging, missing data sets, poor guidance/requirements, unintended outcomes.
If it’s useful people will ask for more intelligence and they will consistently ask more often. I use this premise to understand if the first iterations of what I’ve built is useful. This sentiment is what will lead to consistently greater impact over time.
The sample below demonstrates the types of problems a standardized data enrichment process can solve easily, quickly, and consistently. The graph below details full year results of four customer acquisition channels, three digital, one direct mail. the graph below it calls out the areas of success and the areas of concern. We’ve all seen similar graphs to the one displayed below. However not all companies have analyzed the details as to why they’ve had success or failure at certain points. it would be great to have an auto-generated report that is delivered alongside a graph like this that has the details on why we saw major spikes in certain months and why we saw major drops in others. If we had this info at the same time of the incident occurring, we could take action faster and in the moment. that’s what standardized data enrichment allow us to do. We simply have these standardized processes running in parallel to the data be observed and awe have actionable insights at the moment of impact. Allow us to replicate success where possible and avoid or reduce failure as it occurs.
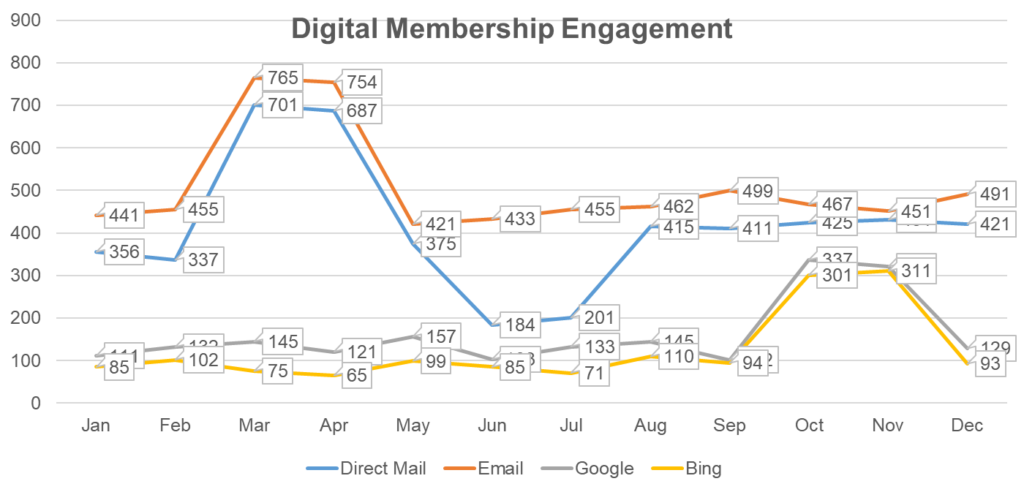
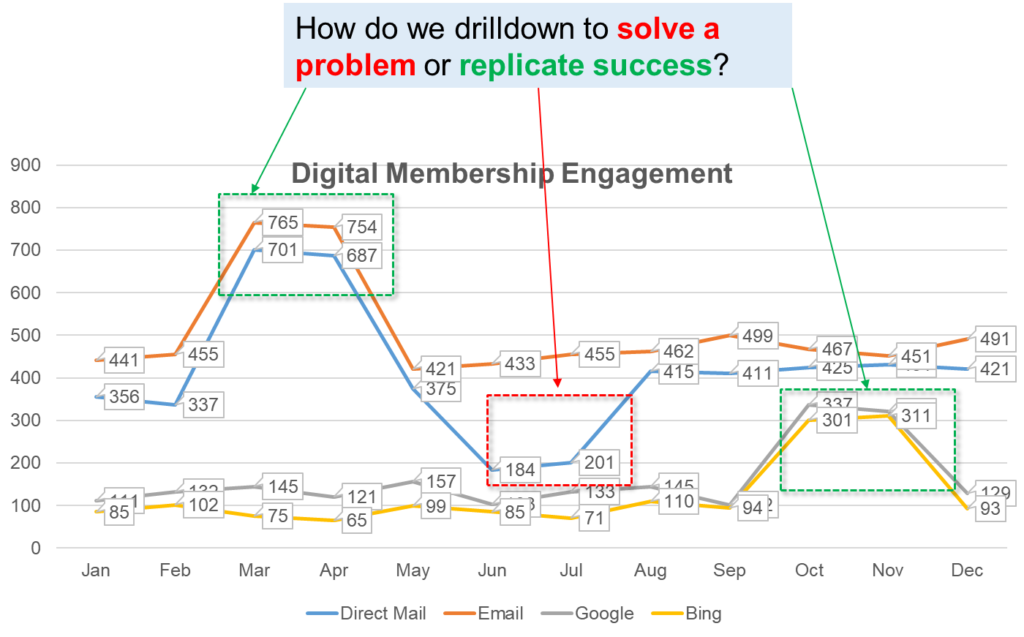
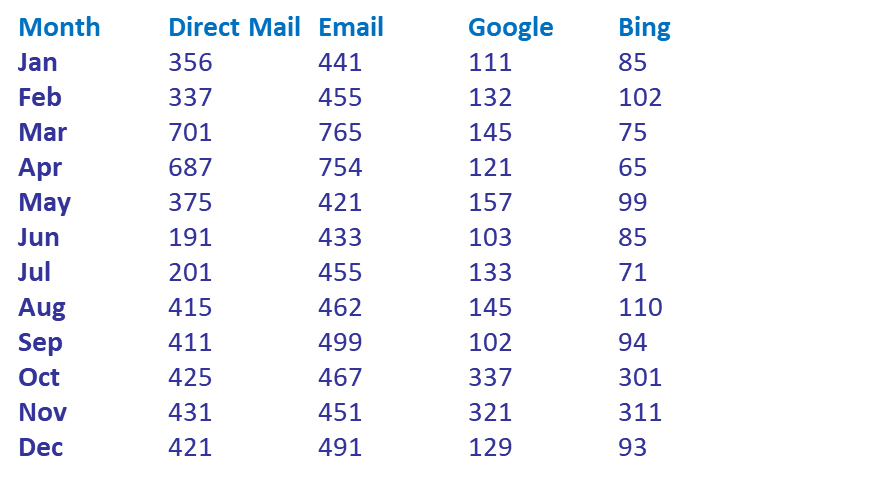